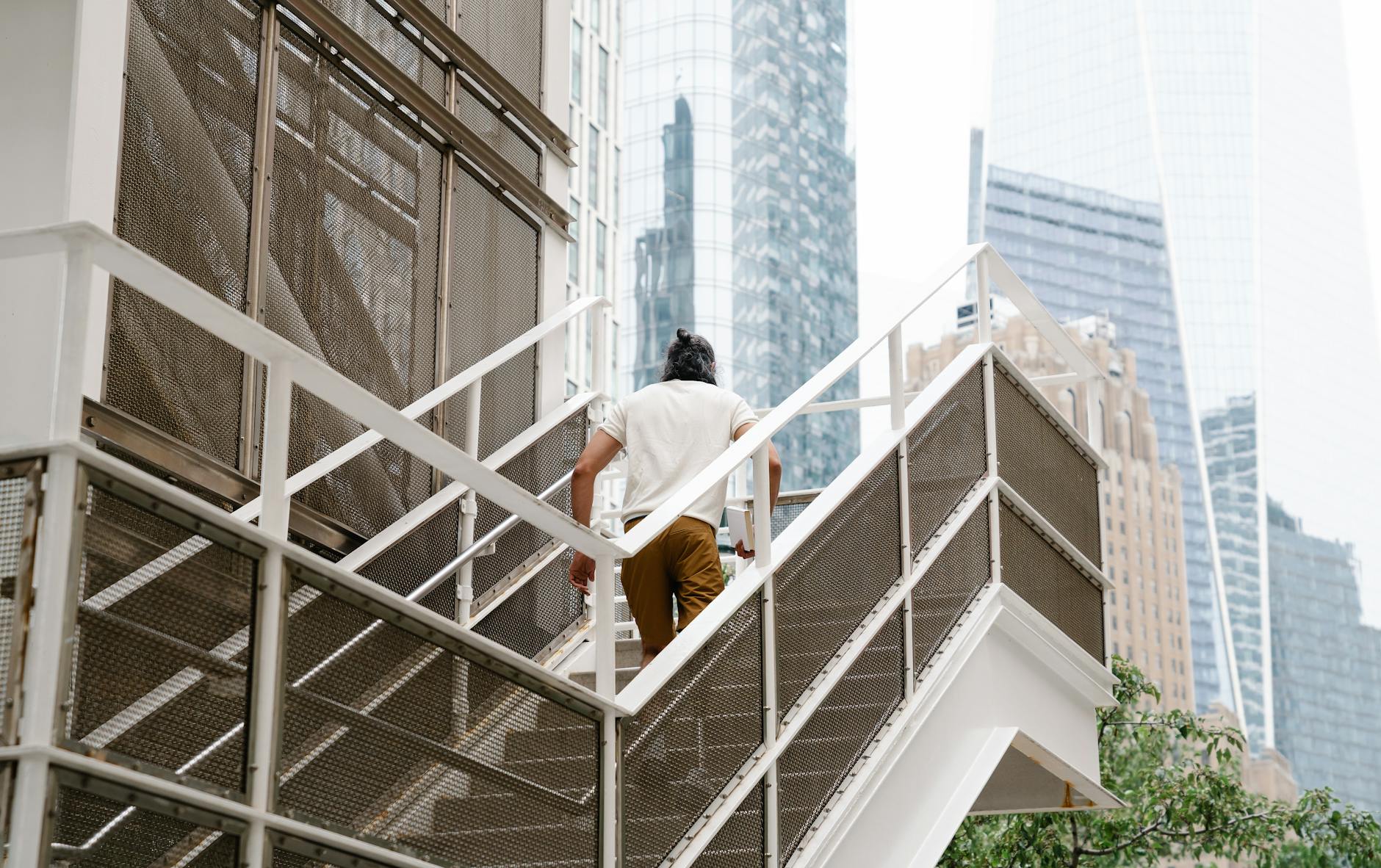
Preparing for AI-Driven Underwriting Skills You Should Highlight
Embracing AI for Streamlined Underwriting: An Industry Overview
Artificial intelligence is transforming underwriting with unprecedented speed, empowering insurance providers to make rapid decisions, achieve more precise risk evaluations, and optimize workflows. For today’s underwriters, this evolution presents an opportunity to go beyond traditional processes, as data analytics and machine learning swiftly transition from niche tech domains to mainstream insurance operations. Embracing AI not only enhances risk analysis and decision-making but also positions insurance professionals as forward-thinking contributors in a field where data-driven strategies increasingly govern success.
Amid this shift, underwriters must adopt new ways of thinking about data. Having just enough familiarity with a new platform is no longer sufficient; genuinely thriving in AI-driven underwriting demands a deeper grasp of how raw information is captured, analyzed, and converted into actionable insights. The ability to communicate effectively with data scientists and other technical teams becomes an essential skill, ensuring that advanced models blend seamlessly with client-centric evaluations. In an arena where machine learning can interpret massive datasets in seconds, a collaborative mindset, soft skills, and commitment to ongoing learning will set standout underwriters apart from the crowd.
As you explore the following sections, you’ll discover the specific competencies that today’s underwriters should cultivate. From understanding emerging data sources and validating the accuracy of datasets to mastering predictive analytics and balancing human insight against AI-generated conclusions, these skills build a strong foundation in an environment where innovation, precision, and ethics rule.
Strengthening Data Literacy for Enhanced Risk Assessment
Modern underwriting success hinges on a solid command of data—where it originates, how it is processed, and why it matters to risk profiles. In the age of AI, data literacy gains new urgency: the more adept underwriters are at interrogating and interpreting data, the more accurately they can align coverage with real-world needs.
Leveraging Modern Data Sources for Strategic Insights
Contemporary risk analysis extends far beyond traditional inputs like credit scores, claims history, and demographic factors. Insurance providers are now incorporating social media metrics, satellite imagery, and sensor-generated data from Internet of Things (IoT) devices to capture nuanced views of any given risk. For instance, a property underwriter may study satellite data to gauge floodplain changes or use IoT-derived readings from smart meters to understand day-to-day operational hazards.
To excel, underwriters should:
- Develop a familiarity with unconventional data streams: By recognizing the impact of emerging sources—such as connected car telematics or social channels—underwriters can propose coverage terms that anticipate highly granular risks.
- Prioritize data relevance: Distinguish between high-level indicators (e.g., real-time building occupancy data) and inconsequential clutter (e.g., irrelevant social posts) to focus on meaningful factors.
- Connect insights to policy decisions: Transform raw data into clear recommendations that strengthen risk management strategies and support accurate premium pricing.
Quality Checks and Validation Protocols for Accurate Risk Profiles
Even the most powerful AI model can falter if data contains inaccuracies or distortions. Duplicated entries, incomplete records, and outdated information can skew algorithms—ultimately leading to poor underwriting decisions. Underwriters who proactively validate the quality of data become invaluable guardians of reliable AI outcomes.
Consider these best practices:
- Cross-reference critical data points: Use multiple data sets or sources to verify suspicious entries and minimize the risk of accepting flawed information.
- Perform regular spot checks: Periodic audits of key metrics help detect anomalies before they cascade into large-scale inaccuracies.
- Collaborate on data governance policies: Work closely with data management teams to establish guidelines that preserve ethical, high-quality datasets, fostering a culture of accountability within the organization.
Leveraging Predictive Analytics to Elevate Underwriting Precision
With AI at the forefront, predictive analytics has become a pillar of underwriting innovation. By harnessing advanced models, insurers can detect patterns that might otherwise remain hidden, anticipate future scenarios, and adjust coverage decisions well before a claim arises. The key lies in using these insights judiciously—ensuring they augment human judgment rather than supplant it.
Maximizing Machine Learning Models for Forward-Looking Insights
Machine learning extends the scope of risk analysis beyond historical trends to include probabilities of future incidents. For example, a commercial automotive insurer might analyze telematics data to predict driver behavior and accident rates, enabling more dynamic pricing. However, the efficacy of such models depends on continuous monitoring and refinement.
Underwriters can strengthen machine learning outcomes by:
- Understanding the fundamentals of algorithmic training: Familiarity with basic principles—like test data, model accuracy, and feature selection—cultivates a well-rounded perspective on model strengths and limitations.
- Engaging with data science professionals: Frequent discussions around model architecture, data transformations, and update schedules build mutual trust and ensure outputs align with an insurer’s overarching risk appetite.
- Requesting transparent model explanations: Opt for approaches like explainable AI (XAI), where possible, to validate how the model arrives at its predictions and guard against unintended biases.
Deploying Predictive Scores for Proactive Interventions
Predictive models often generate risk or health scores that highlight potential problems. These scores empower underwriters to decide when deeper analysis is vital, thereby saving time and resources on more straightforward, lower-risk cases.
For example:
- Fast-tracking standard cases: When an application is flagged as low-risk, underwriters can accelerate policy approvals, boosting efficiency and customer satisfaction.
- Allocating extra scrutiny to outliers: High-risk or anomalous scores may necessitate forensic-level research—e.g., verifying unusual financial data—or seeking additional policy endorsements.
- Recalibrating premiums and terms: When scores reveal an imminent shift in risk exposure, adjusting coverage swiftly translates to a more resilient underwriting portfolio.
Balancing Automation with Human Expertise in AI-Driven Underwriting
While AI automates tasks such as data gathering, risk scoring, and even preliminary underwriting decisions, human insight remains essential for complex or novel risks. Seasoned underwriters can interpret nuances that standardized models may overlook—like subtle behavioral shifts in emerging sectors or context-specific client needs that algorithms have not yet been trained to identify.
Pinpointing Intuitive Judgment for Complex Scenarios
Automated tools excel in routing straightforward applications or identifying black-and-white risks. However, borderline industries—such as new ventures with unproven track records—may confound a purely algorithmic approach. Human underwriters who combine data-driven results with a situational assessment are well poised to:
- Recommend exceptions for innovative businesses: Emerging technologies or services may lack precedent data, but underwriters taking a calculated leap can foster insurer profitability in untapped markets.
- Spot unconventional trends: Shifts in consumer behavior, regulatory frameworks, or local market disruptions might elude machine learning methodologies focused on historical patterns.
- Apply creative policy structures: Tailored risk mitigation strategies, specialized riders, or exclusive coverage clauses can be designed when underwriters have the flexibility to adapt to unique contexts.
Establishing Collaborative Workflows with Technical Teams
Effective AI-driven underwriting emerges from close collaboration among underwriters, data scientists, product managers, and IT professionals. When these groups communicate openly, they can align on key metrics, integrate real-world underwriting concerns into model development, and establish robust processes to handle exceptions without compromising speed.
To build productive alliances:
- Attend cross-functional meetings regularly: Share underwriting experiences that might inform how software updates or new data feeds can be optimized.
- Champion a user-centric approach: Encourage technical teams to incorporate user-friendly dashboards or clear reporting features so underwriters can act on insights swiftly.
- Document your feedback loop: Submit consistent, detailed feedback on model results or application workflows, helping to fine-tune future enhancements.
Ensuring Ethical and Regulatory Compliance in AI-Based Processes
With AI systems increasingly shaping eligibility, pricing, and claims decisions, insurers face heightened scrutiny to ensure fairness and transparency. Regulatory bodies worldwide are imposing stricter guidelines to prevent discriminatory outcomes and protect consumer rights. Underwriters who keep pace with these developments position themselves as ethical gatekeepers and trusted advisors to leadership.
Promoting Transparency and Fairness in Algorithmic Decisions
Biased inputs or opaque algorithms can inadvertently marginalize certain groups or amplify discriminatory practices. Whether it’s factoring in social media data that misrepresents certain communities or overrelying on historical datasets with systemic biases, vigilance is paramount.
Key actions include:
- Routine audits of AI outputs: Examine patterns in policy approvals, premium levels, or claim denials to detect skewed results.
- Adopting explainable AI mechanisms: Prioritize AI setups that provide traceable decision trails, ensuring potential red flags are identified early.
- Advocating for responsible data use: Recognize the difference between predictive correlations and causative factors to avoid penalizing applicants unjustly.
Staying Current with Evolving Insurance Regulations
As technology-driven underwriting gains momentum, new and revised regulations are emerging to address data privacy, consumer protection, and machine learning oversight. Remaining compliant is as much about understanding the legal framework as it is about ethical responsibility.
Best practices include:
- Monitoring regulatory updates: Review policy statements from agencies such as the National Association of Insurance Commissioners (NAIC) or similar bodies in your jurisdiction.
- Aligning with data privacy standards: Policies like the General Data Protection Regulation (GDPR) in Europe may guide how personal information is collected and processed.
- Partnering with compliance experts: Collaborate with in-house or external specialists to integrate legal considerations into your daily underwriting workflow.
Investing in Lifelong Learning and Professional Development
AI’s evolution in the insurance sector shows no sign of slowing. New data streams, advanced analytics tools, and shifting regulations demand that underwriters remain agile and proactive in updating their knowledge. Continuous education fuels personal career growth and ensures an organization retains a competitive edge.
Exploring Specialized Training and Certification Options
Structured courses, workshops, and industry-recognized certifications can sharpen an underwriter’s mastery of data science, analytics, or AI ethics. For instance, programs that delve into statistical modeling or algorithmic interpretability provide deeper context for day-to-day underwriting decisions.
Consider the following:
- Pursue reputable online platforms: Platforms offering certifications in AI and machine learning can strengthen your résumé and help you tackle complex scenarios with greater confidence.
- Seek insurance-specific designations: Bodies like The Institutes or LOMA may offer tailored certificates that integrate underwriting, compliance, and emerging technological innovations.
- Leverage on-the-job training: Many insurers provide internal workshops or subsidize external courses, bridging gaps between theoretical concepts and real-world underwriting challenges.
Expanding Thought Leadership and Networking Opportunities
The robust exchange of ideas in the insurance sector remains critical for fostering innovation. Engaging with peers, mentors, and industry trailblazers not only deepens your understanding of AI-driven strategies but also offers fresh perspectives on tackling underwriting complexities.
To cultivate a strong professional network:
- Attend industry conferences and webinars: Sessions often highlight best practices, case studies, and emerging tools for predictive analytics, data governance, and customer-centric policy design.
- Join relevant online communities: Platforms like LinkedIn groups or specialized forums let you discuss practical AI solutions and gather expert feedback on your underwriting approach.
- Share your expertise: Contribute articles, participate in panel discussions, or post detailed analyses on ongoing challenges—doing so positions you as a knowledgeable, future-ready professional.
Securing Your Future in Advanced Underwriting
As underwriting continues to embrace AI-driven tools, the need for multifaceted skills grows. Underwriters who adapt to new data sources, develop systematic validation processes, and adeptly apply machine learning insights will excel in a technology-centered landscape. Cultivating the ability to balance automation with human discernment—particularly for challenging or emerging risks—also helps insurers maintain a strategic advantage.
From mastering the intricacies of predictive analytics to upholding ethical and regulatory standards, the next generation of underwriting professionals plays a pivotal role in shaping an industry focused on fairness, accuracy, and responsive customer solutions. By taking proactive steps—such as pursuing specialized education, engaging in interdisciplinary collaboration, and vigilantly monitoring legal guidelines—underwriters can establish themselves as indispensable allies to both insurers and policyholders.
General Search & Recruitment stands ready to connect forward-thinking underwriters with organizations eager to lead in an AI-driven marketplace. Through our expertise in talent acquisition and our understanding of insurance industry trends, we help match professionals who possess the vision, technical acumen, and ethical grounding needed to thrive in today’s evolving underwriting landscape.